Machine Learning Innovations in Predictive Maintenance: Strategies and Case Studies for 2025
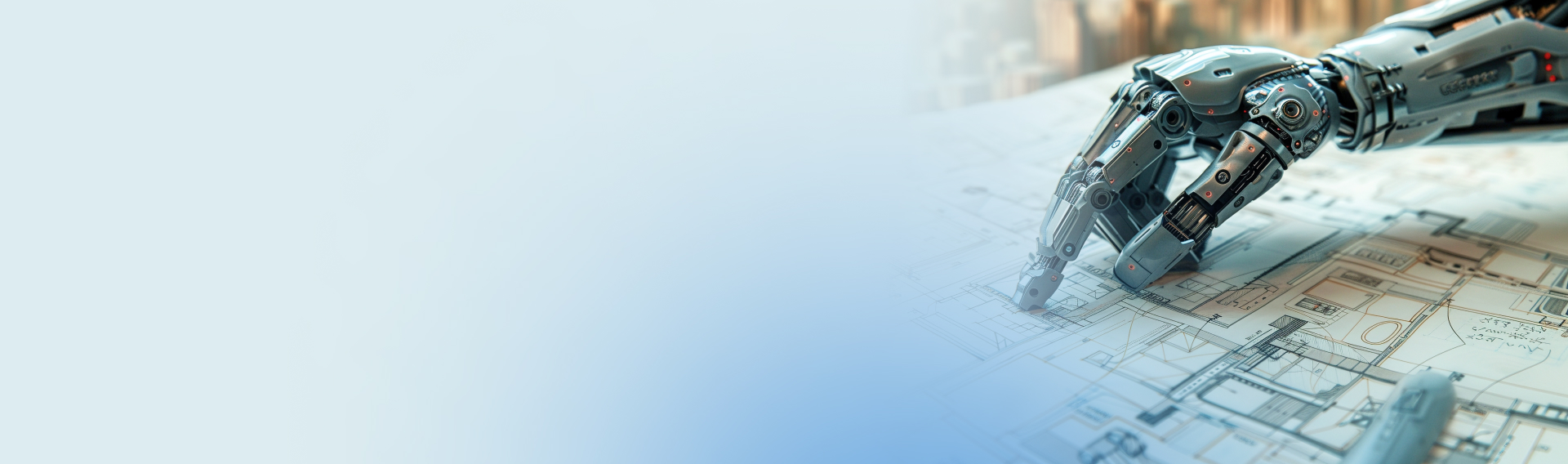
In this article, you will learn what your business can gain from predictive maintenance with machine learning and how it works. Read our tips on implementing this approach to improve operations and prepare for multiple obstacles. Have any questions? Our experts are always here to help.
Reading time: 17 min.
Predictive maintenance first emerged in the crucible of heavy industry, where downtime spelled disaster and towering costs. The cost of machine downtime for the manufacturing and automotive industries can reach $260,000 per hour.
Today, it’s no longer a luxury but a critical strategy for any business looking to outpace competitors and earn customer loyalty. Why does this matter? What must you understand about the essence of predictive maintenance? How do you put it into practice? And what benefits await in the long run?
In this piece, IntexSoft tackles these questions and more, offering you a roadmap to secure your operations and fortify your business strategy.
Let’s take the plunge.
What is it about predictive maintenance that many companies are trying to use?
As you can understand from the very beginning, with predictive maintenance (PdM), organizations utilize data and analytics to anticipate failures (for example, in equipment), taking preventive actions to save money and time, avoid unplanned downtime, prevent unexpected breakdowns, improve efficiency, enhance asset durability, and ensure their business is always in top condition.
Look at the statistics below to uncover the prospects of predictive maintenance algorithms over the next 5 years:
A 35.1% growth rate worldwide – impressive, isn’t it? But what’s driving this quantum leap? The answer lies in new technologies that have taken the world by storm. We mean the Internet of Things (IoT), predictive analytics, artificial intelligence (AI), and machine learning (ML). The sweeping changes brought by these technologies are reshaping industries.
Effortlessly integrate both historical and current equipment data into a range of machine learning models. These cutting-edge systems autonomously pinpoint anomalies and prescribe proactive maintenance strategies to prevent operational disruptions.
Learn how AI-driven predictive maintenance can optimize your business outcomes.
Type of Learning | Description | Examples |
Supervised Learning | In the realm of machine learning, models are meticulously trained on datasets where each instance is paired with a corresponding output label. This rigorous process is ideally suited for tasks like classification—think spam detection—and regression, such as forecasting property prices. | The arsenal of techniques employed in this domain is vast, encompassing Linear Regression, Logistic Regression, Decision Trees, Random Forests, Support Vector Machines, and Neural Networks. |
Unsupervised Learning | Certain models face the daunting task of training on unlabeled datasets, compelled to unearth patterns and relationships independently. This method finds its strength in tasks like clustering—take customer segmentation, for instance—and association, such as market basket analysis. | The toolbox for these endeavors includes K-Means Clustering, Hierarchical Clustering, Principal Component Analysis (PCA), and Anomaly Detection. |
Semi-Supervised Learning | There exists an approach that marries a modest portion of labeled data with a vast expanse of unlabeled data during training. This strategy is particularly advantageous when the cost or effort of labeling data is prohibitive. By leveraging this blend, the model can achieve a deeper understanding without the extensive resources typically required. | – |
Reinforcement Learning | There exists a sophisticated model that learns through direct interaction with its environment, receiving feedback in the form of rewards or penalties. This method is deployed in scenarios requiring a series of decisions, such as in game playing or robotics. | Techniques such as Q-Learning, Deep Q-Networks (DQN), and Policy Gradient Methods are instrumental in these complex tasks, driving the model to refine its strategies through continuous adaptation and response. |
Four stages are commonly found in predictive maintenance workflows. Look at the scheme below:
Let’s highlight some essential aspects of each stage individually.
Imagine a business where advanced sensors are embedded in machinery, constantly gathering data on temperature, vibration, pressure, and usage. Take a manufacturing plant, for instance—sensors on the production lines track key metrics like conveyor belt speed, motor temperature, and hydraulic pressure. This information is then sent in real-time to AI platforms in the cloud or on-site systems.
This stage is essential for all industries. We have shown how it begins in manufacturing. Data collection depends on the field, but the primary goal is to gather all information that can impact the result.
AI algorithms process incoming data streams to assess equipment health instantly. For example, in an HVAC system, AI meticulously analyzes temperature trends and air flow rates, predicting potential faults like overheating components or diminished efficiency from clogged filters. When anomalies surface, automated alerts are dispatched to maintenance teams through mobile applications or integrated messaging systems, urging them to investigate and take preemptive action. This seamless integration of technology and foresight epitomizes the future of predictive maintenance.
Machine learning models leverage historical data to forecast equipment performance trends. For instance, in fleet management, ML algorithms can predict engine failures in trucks based on patterns identified from past maintenance records and operational conditions. These insights enable predictive maintenance schedules that optimize part replacements and reduce unplanned downtime, enhancing fleet reliability and operational efficiency.
With each new dataset integrated into the AI system, predictive models undergo continuous refinement. In the energy sector, for instance, AI-driven analytics continuously monitor wind turbine performance data. Over time, these models learn to anticipate maintenance needs based on evolving weather patterns and turbine usage, ensuring optimal performance and extending equipment lifespan.
IntexSoft AI experts have seasoned expertise in implementing various AI solutions. You can confirm this by contacting our team.
Now it’s time to concentrate on two industries that are strongly needed in PdM: ecommerce and telecom.
AI-driven PdM is quietly changing ecommerce, an industry where downtime is the prime enemy. Consider fulfillment centers. AI systems are on constant vigil, monitoring every conveyor belt, sorting machine, or piece of packaging equipment.
But the most valuable benefit is in analyzing. Such tech scrutinizes data on operational speed, vibration, and wear and tear with an almost relentless precision. The moment they detect an anomaly, systems spring into action. Alerts are sent to maintenance teams instantly, often before human operators even notice there’s an obstacle.
This proactive stance slashes downtime, keeping the machinery of commerce humming smoothly. Orders are processed without delay, packages are shipped on time, and customers remain satisfied, often oblivious to the potential crises that were averted behind the scenes.
Telecom towers, base stations, and data centers are equipped with numerous sensors that monitor various parameters such as temperature, humidity, power consumption, and signal strength. AI algorithms analyze this data to detect patterns indicative of potential equipment failures.
For example, a slight increase in temperature within a server rack might indicate an impending cooling system failure. By predicting such issues, telecom companies can perform maintenance activities during off-peak hours, minimizing service disruptions and ensuring continuous connectivity for customers.
The driving force behind adopting predictive maintenance must be clear: enhancing operational efficiency, minimizing downtime, and achieving financial savings. To reach these objectives, crafting a detailed implementation strategy is essential. Here’s a comprehensive outline to guide this process.
The first thing to remember is that the quality, relevance, and consistency of the data are paramount, serving as the bedrock for effective machine learning models. These models, in turn, promise improved equipment reliability, reduced downtime, and substantial cost savings.
Businesses must confront several challenges head-on. Imbalanced datasets, data drift, model interpretability, and scalability issues often stand in the way of smooth implementation.
Addressing these hurdles requires a strategic approach. Techniques like oversampling or undersampling can balance datasets. Continuous monitoring is essential to detect and manage data drift, ensuring that models remain accurate over time.
Implementing PdM isn’t a set-it-and-forget-it solution. Constant monitoring of equipment conditions and performance is necessary for timely interventions. This is where new data plays a pivotal role, allowing machine learning models to be updated and continuously optimized, thereby enhancing the effectiveness of predictive maintenance efforts.
Now that you’ve grasped the essence of AI and predictive maintenance, crucial considerations for today’s business owners and C-level executives, let’s emphasize the key factors to evaluate when establishing PdM.
Refer to the list below:
To receive a free consultation from our seasoned experts regarding any questions or specific issues about predictive maintenance machine learning, click “Contact Us.”